-
Notifications
You must be signed in to change notification settings - Fork 0
/
README.qmd
355 lines (296 loc) · 12.1 KB
/
README.qmd
1
2
3
4
5
6
7
8
9
10
11
12
13
14
15
16
17
18
19
20
21
22
23
24
25
26
27
28
29
30
31
32
33
34
35
36
37
38
39
40
41
42
43
44
45
46
47
48
49
50
51
52
53
54
55
56
57
58
59
60
61
62
63
64
65
66
67
68
69
70
71
72
73
74
75
76
77
78
79
80
81
82
83
84
85
86
87
88
89
90
91
92
93
94
95
96
97
98
99
100
101
102
103
104
105
106
107
108
109
110
111
112
113
114
115
116
117
118
119
120
121
122
123
124
125
126
127
128
129
130
131
132
133
134
135
136
137
138
139
140
141
142
143
144
145
146
147
148
149
150
151
152
153
154
155
156
157
158
159
160
161
162
163
164
165
166
167
168
169
170
171
172
173
174
175
176
177
178
179
180
181
182
183
184
185
186
187
188
189
190
191
192
193
194
195
196
197
198
199
200
201
202
203
204
205
206
207
208
209
210
211
212
213
214
215
216
217
218
219
220
221
222
223
224
225
226
227
228
229
230
231
232
233
234
235
236
237
238
239
240
241
242
243
244
245
246
247
248
249
250
251
252
253
254
255
256
257
258
259
260
261
262
263
264
265
266
267
268
269
270
271
272
273
274
275
276
277
278
279
280
281
282
283
284
285
286
287
288
289
290
291
292
293
294
295
296
297
298
299
300
301
302
303
304
305
306
307
308
309
310
311
312
313
314
315
316
317
318
319
320
321
322
323
324
325
326
327
328
329
330
331
332
333
334
335
336
337
338
339
340
341
342
343
344
345
346
347
348
349
350
351
352
353
354
---
format: gfm
---
This repo contains ideas, code, and example data to estimate 'cyclability' on transport networks.
At present it is focussed on cycling because there are more readily available datasets for cycling than for walking that we are aware of.
However at some point we would like to extend this to walking and other modes, in which case the repo may be renamed (e.g. to `activability` if nobody comes up with a better name ; ).
## What is cyclability?
There are at least three definitions of how conducive to
cycling different places, routes and segments of travel networks are:
- [Level of Traffic
Stress](https://docs.conveyal.com/learn-more/traffic-stress) (LTS)
- [Bikeability](https://www.britishcycling.org.uk/cycletraining/article/ct20110111-cycletraining-What-is-Bikeability-0)
levels, which rates infrastructure based on the level of training
needed to feel comfortable:
- Level 1 teaches basic bike-handling skills in a controlled
traffic-free environment.
- Level 2 teaches trainees to cycle planned routes on minor roads,
offering a real cycling experience.
- Level 3 ensures trainees are able to manage a variety of traffic
conditions and is delivered on busier roads with advanced features
and layouts
- CycleStreets’s [Quietness
rating](https://www.cyclestreets.net/help/journey/howitworks/#quietness)
from 1 (very unpleasant for cycling) to 100 (the quietest)
- The [BNA tool](https://bna.peopleforbikes.org/#/) which builds on the concept of traffic stress to classify segments as Low Stress or High Stress.
## Example data
### Data from Leeds, UK
Datasets containing estimates of 'quietness' and 'cyclability' for Leeds, UK, are available from the a separate [repo](https://github.com/ITSLeeds/cyclability/).
These datasets were taken from an area with the following bounding box:
```
xmin ymin xmax ymax
-1.571467 53.797790 -1.541108 53.815759
```
This area, representing a 1 km boundary around the University of Leeds (-1.556288, 53.80677) can be seen in OSM at the following URL: https://www.openstreetmap.org/#map=16/53.8068/-1.5563
To generate a GeoJSON version of the input data we used the `osmtogeojson` command line utility which can be installed on Ubuntu with the following command:
```bash
sudo npm install -g osmtogeojson
```
#### OSM data
```{r}
#| echo: false
#| eval: false
leeds_university = tmaptools::geocode_OSM("university of leeds", as.sf = TRUE)
leeds_smallarea = stplanr::geo_buffer(shp = leeds_university, dist = 1000)
dir.create("example-data")
leeds_bounding_polygon = stplanr::bb2poly(sf::st_bbox(leeds_smallarea))
sf::write_sf(leeds_bounding_polygon, "example-data/leeds_bounding_polygon.geojson")
sf::st_bbox(leeds_smallarea)
```
OSM data was downloaded from overpass with the following command which uses `wget` to query the API for the bounding box:
```bash
#| eval: false
wget -O example-data/leeds.osm "https://overpass-api.de/api/interpreter?data=[out:xml][timeout:25];(way[highway](53.797790,-1.571467,53.815759,-1.541108);node(w););out body;>;out skel qt;"
```
The output of the command above can be found in the `example-data` folder of this repo.
```{bash}
#| eval: false
osmtogeojson example-data/leeds.osm > example-data/leeds.geojson
```
<!-- Convert this to GeoJSON with the following command (requires `osmium-tool`): -->
```{bash}
#| echo: false
#| eval: false
osmium cat -o example-data/leeds.geojson example-data/leeds.osm
```
```{r}
#| echo: false
#| eval: false
# Run with osmextract: https://github.com/ropensci/osmextract/issues/281
remotes::install_dev("osmextract")
leeds_osmextract = osmextract::oe_read("example-data/leeds.osm")
```
The first 10 keys in the OSM dataset that have at least one value are:
```{r}
#| warning: false
#| echo: false
library(tidyverse)
leeds_osm_osm = sf::read_sf("example-data/leeds.osm", layer = "lines")
leeds_osm_geojson = sf::read_sf("example-data/leeds.geojson")
leeds_osm_names = names(leeds_osm_geojson)
leeds_osm_names[1:10]
# nrow(leeds_osm_geojson)
# nrow(leeds_osm_osm)
```
The OSM data can be seen in the map below:
```{r}
#| echo: false
plot(sf::st_geometry(leeds_osm_osm))
```
The example dataset contains around 3k line segments and 200 keys with at least one value.
The number of features by geometry type is shown in the table below.
```{r}
#| echo: false
leeds_osm_geojson |>
mutate(geometry_type = sf::st_geometry_type(leeds_osm_geojson)) |>
sf::st_drop_geometry() |>
count(geometry_type) |>
knitr::kable()
```
#### Quietness
A GeoJSON file with quietness estimates for each road segment in Leeds is available at https://github.com/ITSLeeds/cyclability/raw/main/cyclestreets/leeds_quietness.geojson and is illustrated below:
```{r}
#| echo: false
leeds_quietness = sf::read_sf("https://github.com/ITSLeeds/cyclability/raw/main/cyclestreets/leeds_quietness.geojson")
plot(leeds_quietness["quietness"])
leeds_quietness |>
sf::st_drop_geometry() |>
dplyr::slice(1:3) |>
knitr::kable()
```
A simple model was used to find out the relationship between the quietness rating and riding surface, resulting in the following plot:
```{r}
#| echo: false
model_lm = lm(quietness ~ ridingSurface, data = leeds_quietness)
# Get the R squared value:
model_lm_rsquared = summary(model_lm)$r.squared
model_tidy = broom::tidy(model_lm, conf.int = TRUE)
intercept = model_tidy |>
dplyr::filter(term == "(Intercept)") |>
dplyr::pull(estimate)
# Visualise the resulting model with ggplot2:
# model_tidy$term = as.factor(model_tidy$term)
# levels(model_tidy$term) = model_tidy$term[order(model_tidy$estimate)]
# model_tidy$term = ordered(model_tidy$term)
```
```{r surface-quietness, fig.width = 6, fig.height = 4}
#| echo: false
model_tidy |>
dplyr::filter(term != "(Intercept)") |>
# mutate(across(matches("est|conf"), function(x) x + intercept)) |>
mutate(term = gsub("ridingSurface", "", term)) |>
# ggplot2 call with confidence intervals:
ggplot2::ggplot(ggplot2::aes(x = term, y = estimate)) +
ggplot2::geom_point() +
ggplot2::geom_errorbar(ggplot2::aes(ymin = conf.low, ymax = conf.high)) +
ggplot2::coord_flip() +
ggplot2::labs(
x = "Riding surface",
y = "Quietness rating effect size (low values are less cycleable)"
# ,
# title = "Relationship between riding surface and quietness rating"
)
```
From this we can see that, as would be expected, living streets and pedestrianised areas are associated with the highest quietness ratings.
The model also reveals that, for the sample data in Leeds at least, the segment type alone can explain `r round(model_lm_rsquared * 100)`% of the variation in quietness ratings.
The dependent variables shown in the plot above are produced by CycleStreets which were themselves derived from OSM data.
Let's try to reproduce the quietness ratings using the OSM data directly.
To do that, we first need to join the data from CycleStreets with the OSM data.
The OSM data contains ids such as:
```{r}
leeds_osm_geojson$id[1:3]
```
The CycleStreets data contains ids such as:
```{r}
leeds_quietness$id[1:3]
```
After removing everything before and including the `/` character, the ids are the same, with the majority of the ids in the CycleStreets data present in the OSM data:
```{r}
leeds_osm_geojson$id = gsub(".*\\/", "", leeds_osm_geojson$id)
summary(leeds_quietness$id %in% leeds_osm_geojson$id)
```
```{r}
#| echo: false
# Check to see if the OSM data has the same IDs as the CycleStreets data:
leeds_osm_geojson_df = leeds_osm_geojson |>
sf::st_drop_geometry() |>
# Convert id column to integers:
dplyr::mutate(id = as.integer(id))
leeds_quietness_joined = leeds_quietness |>
select(-name) |>
dplyr::left_join(leeds_osm_geojson_df, by = c("id" = "id"))
```
Of the 200+ keys in the OSM data, only a few appear frequently enough to be useful for modelling.
The keys that most frequently have values are shown in the table below:
```{r}
#| echo: false
all_keys_df = leeds_osm_geojson_df |>
dplyr::select(-id) |>
dplyr::mutate(across(everything(), as.character)) |>
tidyr::pivot_longer(cols = everything()) |>
dplyr::filter(!is.na(value)) |>
group_by(name) |>
dplyr::summarise(
n = n(),
most_common_value = names(which.max(table(value))),
most_common_value_count = max(table(value))
) |>
dplyr::arrange(desc(n))
most_common_keys_df = all_keys_df |>
dplyr::slice(1:20)
most_common_keys_df |>
knitr::kable()
```
Running the same model on the OSM data gives the following results and explains a higher proportion of the variation in quietness ratings: just over 90%.
```{r}
#| echo: false
names_to_keep = c("quietness", "highway", "maxspeed", "bicycle")
leeds_quietness_joined_minimal = leeds_quietness_joined[names_to_keep] |>
# Convert all columns, except quietness, to character:
mutate(across(-quietness, as.character)) |>
sf::st_drop_geometry()
model_lm_osm = lm(quietness ~ ., data = leeds_quietness_joined_minimal)
# Get the R squared value:
model_lm_osm_rsquared = summary(model_lm_osm)$r.squared
# model_lm_osm_rsquared
```
```{r}
#| echo: false
model_tidy = broom::tidy(model_lm_osm, conf.int = TRUE)
intercept = model_tidy |>
dplyr::filter(term == "(Intercept)") |>
dplyr::pull(estimate)
# Visualise the resulting model with ggplot2:
# model_tidy$term = as.factor(model_tidy$term)
# levels(model_tidy$term) = model_tidy$term[order(model_tidy$estimate)]
# model_tidy$term = ordered(model_tidy$term)
```
```{r lm_osm, fig.width = 6, fig.height = 4}
#| echo: false
#| warning: false
model_tidy |>
dplyr::filter(term != "(Intercept)") |>
# mutate(across(matches("est|conf"), function(x) x + intercept)) |>
mutate(term = gsub("highway", "", term)) |>
# ggplot2 call with confidence intervals:
ggplot2::ggplot(ggplot2::aes(x = term, y = estimate)) +
ggplot2::geom_point() +
ggplot2::geom_errorbar(ggplot2::aes(ymin = conf.low, ymax = conf.high)) +
ggplot2::coord_flip() +
ggplot2::labs(
x = "Riding surface",
y = "Quietness rating effect size (low values are less cycleable)"
# ,
# title = "Relationship between riding surface and quietness rating"
)
```
```{r}
#| echo: false
# remotes::install_cran()
# model_brms =
```
```{r}
#| eval: false
#| echo: false
# Predict quietness as a function of ridingSurface with lightGBM
remotes::install_cran("lightgbm")
remotes::install_cran("bonsai")
library(bonsai)
set.seed(2023)
leeds_quietness_df = leeds_quietness |>
sf::st_drop_geometry() |>
dplyr::select(quietness, ridingSurface)
dt_mod = boost_tree() |>
set_engine(engine = "lightgbm") %>%
set_mode(mode = "regression") %>%
fit(
formula = quietness ~ ridingSurface,
data = leeds_quietness_df
)
dt_mod
```
```{r}
#| echo: false
#| eval: false
# Failed lightgbm example:
quietness_model = lightgbm::lightgbm(
quietness ~ ridingSurface,
data = leeds_quietness |> sf::st_drop_geometry(),
objective = "regression",
metric = "rmse",
num_iterations = 1000,
learning_rate = 0.1,
num_leaves = 31,
min_data_in_leaf = 20,
bagging_fraction = 0.8,
bagging_freq = 5,
feature_fraction = 0.8,
early_stopping_round = 10
)
```
## Live examples
### Network Planning Tool
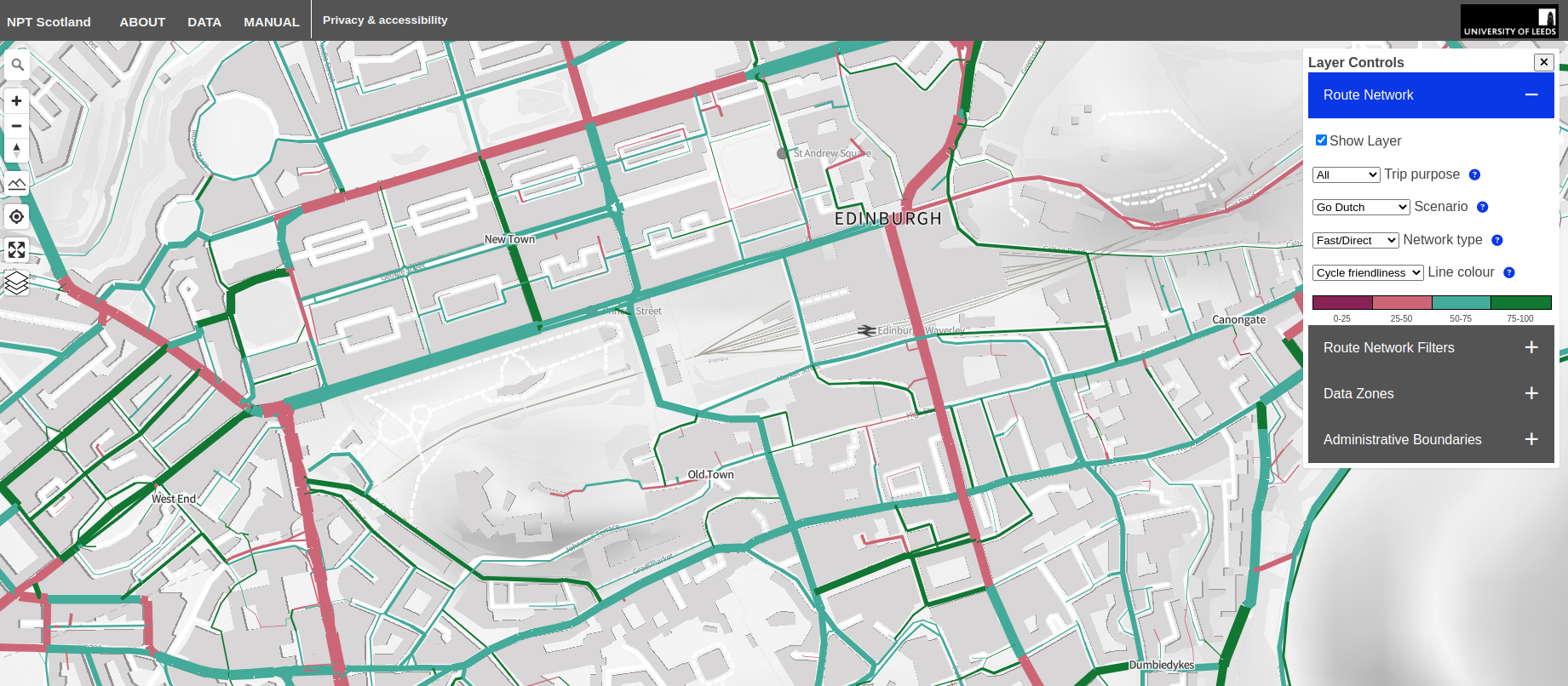
Source: https://nptscot.github.io/#14.75/55.94993/-3.19227
## Prior methodological work and implementations
- The Bike Network Analysis (BNA) tool methodology: https://cityratings.peopleforbikes.org/about/methodology
- Discussion of cyclability in A/B Street issue tracker: https://github.com/a-b-street/abstreet/issues/600
- Gist in Python on GitHub calculating cyclability from OSM: https://gist.github.com/aroche/d6fd03e51869c3e554f908bc14b5750b
- Methods described in by CycleStreets: [Quietness
rating](https://www.cyclestreets.net/help/journey/howitworks/#quietness)
## Thoughts on next steps (draft)
- [ ] Prototype code to generate plausible quietness ratings from OpenStreetMap data
- [ ] Wire up to a web interface
- [ ] Create a frontent to allow people to tweak the parameters affecting cyclability
- [ ] Develop default settings e.g. for different types of users (e.g. novice, experienced, confident) and implementations of different metrics (e.g. Bikeability 1 to 3 or LTS 1 to 4)
- [ ] Encode the settings that lead to these implementations in a human readable and easy-to-edit format, e.g. JSON